Catherine Roy and I, as members of the Multi-Stakeholder Forum to Open Government, Government of Canada, and as Chairs and Co-chairs of the MSF commitment on Data for Results: Disability, submitted the following to the Government of Canada commitments to the international Open Government Partnership. This would be for the next Action Plan For Canada.
Catherine and I officially met with Government of Canada representatives, beyond the MSF officials, on this important commitment, on June 28, 2021. We had requested to meet, as part of the engagement process, with officials from Employment and Social Development Canada (ESDC), and the Canadian Accessibility Standards Development Organization. They unfortunately did not attend the civil society consulation. We did meet with officials from Statistics Canada; the Office of the Deputy Minister of Public Service Accessibility and the Canada Revenue Agency in addition to our usual MSF Open Government Team at the Treasury Board Secretariat of Canada.
The Civil Society Commitments we submitted and discussed, were developed in consultation with civil society disabled people’s organizations, experts and advocates with lived experience. It was the first time that such a group had been brought together to specifically discuss data about disabled people in Canada.
After the meeting, the civil society representatives met, and recommended an additional commitment and refined the 7 point Data For Results on Disability Commitment as posted below, that was presented to government. We also reached out to those who sent their regrets for their input. It was great to have these key experts, people with lived experience and disabled people civil society organizations at the table. This was no small feat given the context of the pandemic and the front line priorities these organizations and people have.
We then formally asked that these Refined Commitments be shared with government of Canada counterparts in an email. In that correspondence we offered to look at a draft, and or to discuss ideas. It is conventional to discuss draft commitments.
Unfortunately, the Government of Canada actors responsible for this commitment did not discuss their proposed commitments prior to the public consultation process, and as you can see here, the Government’s proposal does not resemble what civil society actors requested. The Government’s draft commitment does however resemble the 2021 Federal Budget announcement on disagregated data, and new additions that just came in this afternoon, are a list of work government was already doing, thus these do not consitutue new commitments. It is great that this work is beind done, but these do not address the request that was made. (see below Civil Society Commitments 1 & 2)
As per the process, we formally submitted our disapointment to the Government of Canada proposal in an email, and we did so today again, and we have submitted comments as part of the online consultation process which you can find here and I have posted images below. We hope that this part of the process works, and that our questions and comments will be responded to. You can submit comments to!
We also hope that we will be able to work together on addressing how disabled people are unseen and generally unacounted for in government statistics, government data and administrative data, let alone open data, and that when they are, they are often misclassified or overly surveyed and audited in programs and taxation as they are often identified as outliers. Also, that data will be collected about topics and issues of importance to disabled people, in the spirit of Nothing about us with out us!
We believe the Government operated with good intentions, and we hope to be able to work together to fulfill the requests from civil society and, that our commitments will become policy and that we can have meaningful dialogue to co-create open government policy as per the process.
Preamble
Data for Results: Data about People with Disabilities
MSF Civil Society actors identified a significant data deficit regarding the living conditions and well-being of people with disabilities in Canada. These historical and systemic inequities became more visible and problematic throughout COVID-19 pandemic response. Existing data are outdated, insufficient, difficult to find and do not address systemic issues.
People with disabilities are counted as part of the Employment Equity Act in terms of employment in the Federal Government, there is a new Act to ensure a barrier-free Canada, which has led to the creation of a Canadian Accessibility Standards Development Organization (CASDO), the Canadian Survey on Disability (CSD) targets people who are 15 years and over on Census day. These are to be lauded, but there remains an absence of data about the living conditions of this group of people in terms of individual residences and most especially collective dwellings which more often than not involves state support. There is also a lack of any national understanding of how this group of people in Canada are faring and when in collective dwellings, how much freedom is afforded in terms of movement. This would be the responsibility of Statistics Canada, the CASDO, and the Public Health Agency of Canada (PHAC).
Currently there is no one statistical unit responsible for the collection of data, surveys and administrative data about people with disabilities. There is no national dataset nor a typology of collective residences and collective care facilities such as group homes, home care, and respite care. There are no unique classification systems, and the General Social Survey and the Census do not survey people with disabilities where they live, missing those who live in institutions and custodial housing. We also know little of the employment, education, health, and economic status and wellbeing of this group of Canadians. This is a first step to knowing more, and to inform policies, programs and services. Internationally this group of people are underrepresented, invisible and unseen in Open Data priorities. We hope to also address this international Open Government and Open Data gap.
Multi-Stakeholder Forum Civil Society Commitment Requests on the topic of Open Data For Results – Disability:
Data for Results – Disability Civil Society Commitment 1:
• We would like the following 7 step Data For Results process on Disability commitment:
1. Disability Data Inventory – Conduct a cross government of Canada inventory to identify data, surveys, administrative data, program and service data, models, crowdsourcing data collection programs about disabled people, including their social and material lived experience. This would include methodological guides, data dictionaries, classification systems & taxonomies, standards, etc. as well as data owners, and how the data are used in decision making and reporting.
2. Analyze – Analyze the inventory and identify data gaps and issues with disability civil society actors and experts in and outside of government. This includes identifying risks related to automated decision making, validity of the data, assessment of proxies, bias and models of disability. Also, with great attention to the potential ramifications of data to the lives of disabled people i.e. disabled people are often audited more by the CRA as their lives do not follow traditional life courses.
3. Publish – The results of the inventory and later the analysis of the inventory on the Open Government Website and publish any data that can be made open in the open data portal with accompanying documentation. This can be a type of clearinghouse on disability, including laws, regulations, official reporting, etc.
4. Collect – Develop procedures to ameliorate existing datasets, and work with Civil Society actors and experts in and outside of government to produce and collect new data with responsible authorities, keeping in mind the risks discussed earlier.
5. Publish – New, current, and historical existing data on the Government of Canada Open Data portal and also, for those data that cannot be open data, these ought to be listed with details and a contacting authority.
6. Act – Data for Results is about informing public policy, programs and services to address inequities and the social and material conditions of disabled people, to assess impact and outcomes of regulation, to mitigate ableism and to improve data collection, dissemination and processes including impact and oversight, while also creating affirmative indicators related to participation in Canadian life and the workforce. Impact metrics will be required to monitor progress.
7. Report Results – Report policy, program and service improvements and outcomes.
Data for Results – Disability Civil Society Commitment 2:
Considering the importance of the availability of data designed according to and compatible with the systemic social model of disability to ensure the monitoring of the Convention on the Rights of Persons with Disabilities (UNCRPD) ratified by Canada, it is recommended to include the Human Rights Commission of Canada in any discussion related to the government’s commitment to open data in the area of disability. In addition, the lead Canadian organization in monitoring the CRPD with civil society partners is the British Columbia Aboriginal Network on Disability Society (BCANDS) crpd@bcands.bc.ca “
Image of the Government of Canada Response to our Initial Request and what is currently out for consultation:
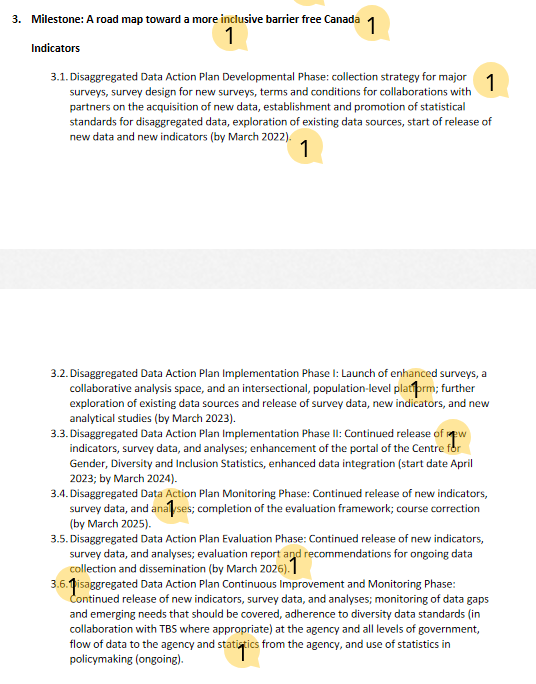
Update – Wereceived notice at 14:00 YOW time, that the following was forgottent in the Government Consulation Document
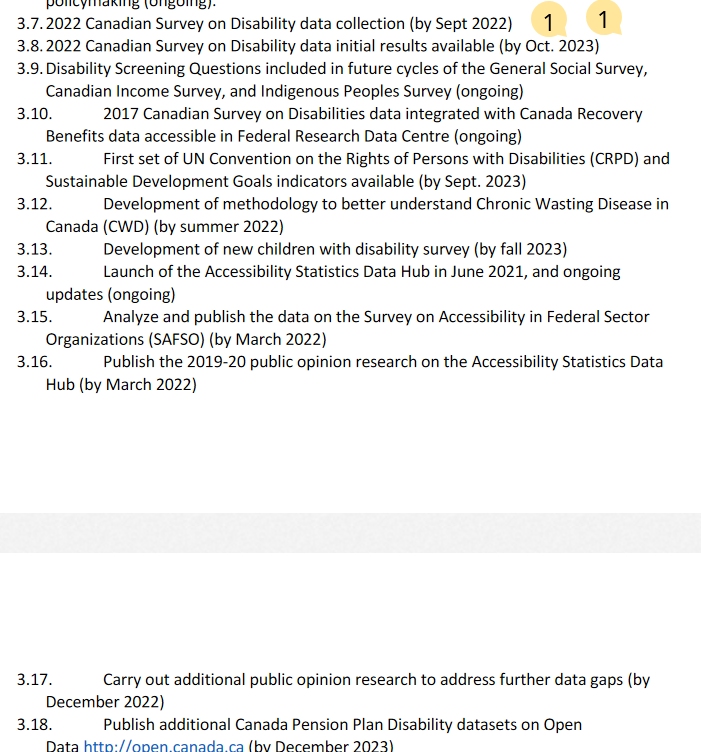
My submitted comments about this oversight are as follows:
“The addition of 3.7 onward, just now on a Friday afternoon before an election, is great, but these do not constitute Open Government Commitments, as these are the usual business of Government, some of the surveys should not have been cancelled in the first place, and these were things Government had already committed to. We are glad that you are going to do them. Also they have just been added on the last days of the consultation. In addition, some of these surveys do not sample disabled people living in collective dwellings such as domiciliary institutions, the living arrangements for many disabled people, which means it will miss many resulting in a gross under count and under representatio, especially people with developmental disabilities and potentially the elderly with disabilities who live in elder care homes, and any other disabled people relegated to prisons because of inadequate mental institutions, and others with developmental disabilities in mental institutions because of a lack of other forms of care.
We of course, continue to hope that our group of civil society actors will be consulted and an advisory board will be constituted with the people and organizations who helped shape and endorse the civil society submitted commitments.
Part of the issues identified by civil society, are these types of data gaps in existing counting systems, including a clear lack of typologies and classification systems about foundational issues such as living arrangements and dwelling types.
Finally, as is the case with Indigenous people, there ought to be systems developed by and with disabled people as the current deficit indicators are not useful, and what of data that are meaningful to disabled people?
Again, we are most willing to work with you. And we hope that our call for an inventory, the analysis of the data from that inventory, and an advisory group can be set up in an official capacity so that we do this work together. We look forward to your thoughts on this. We have posted our observations and requests here as comments to this document and also here https://www.datalibre.ca/2021/08/13/open-data-for-results-disability/.
Also note, that the groups we worked with as part of this process, expected their contribution in terms of the development of commitments to be enough, they did not expect to have to come back to a website and also have to reiterate what they have already share with you. Thus we did!
Again, we believe the Government has good intentions, but in the absence of dialogue with us, missed what we were proposing and why. The Open Government process involves meaningful engagement, and new commitments beyond usual government business. I am sure we will be able to help you formulate a commitment that is new, and one that is meaningful to disabled people in Canada.”
Comments on Posts